Create Your First Project
Start adding your projects to your portfolio. Click on "Manage Projects" to get started
Bearing Fault Detection and Classification using Signal Processing and Machine Learning
Project type
Mechanical Design, AI/ML
Date
Oct 2020 - Jan 2021
Skills
Machine Learning · Signal Processing · MATLAB
This project focuses on detecting and classifying faults in ball bearings using a combination of signal processing techniques and machine learning models. The dataset includes vibration signals collected from an induction motor-driven system under different fault conditions. Key methods include pre-processing, feature extraction using techniques like Hilbert Transform, and the application of machine learning algorithms to classify bearing health conditions.
Key Features:
- Signal Pre-processing: Applied filtering and noise reduction techniques to the raw vibration signals for accurate feature extraction.
- Feature Extraction: Utilized advanced methods like Hilbert Transform, Envelope Analysis, and Morphological Analysis to capture key characteristics of bearing vibration signals.
- Machine Learning Models: Employed models such as Support Vector Machines (SVM), K-means clustering, and decision trees to classify various bearing fault conditions, including outer race, inner race, and ball faults.
- Cross-Validation and Performance Metrics: Used cross-validation techniques to evaluate model performance and ensured high classification accuracy through performance metrics like precision, recall, and F1 score.
- Automated Fault Diagnosis: The system is designed for real-time fault detection and classification, making it suitable for predictive maintenance in industrial applications.



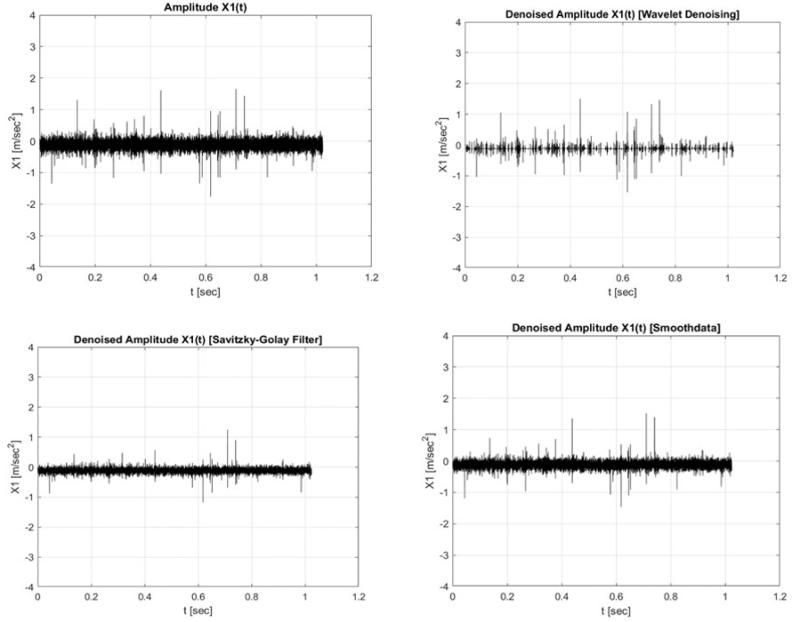



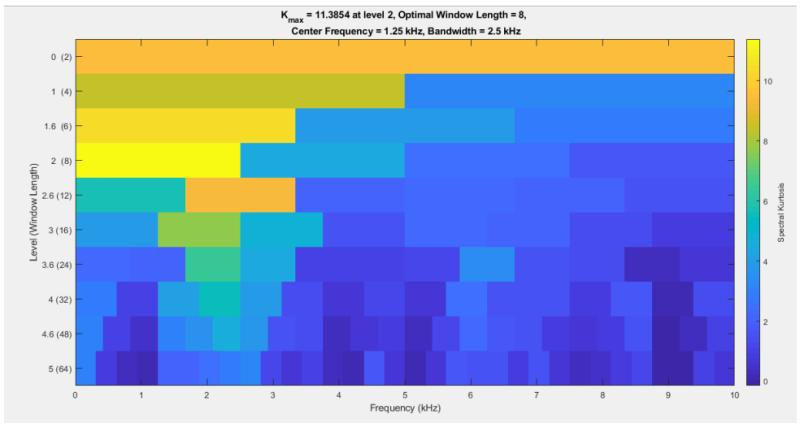

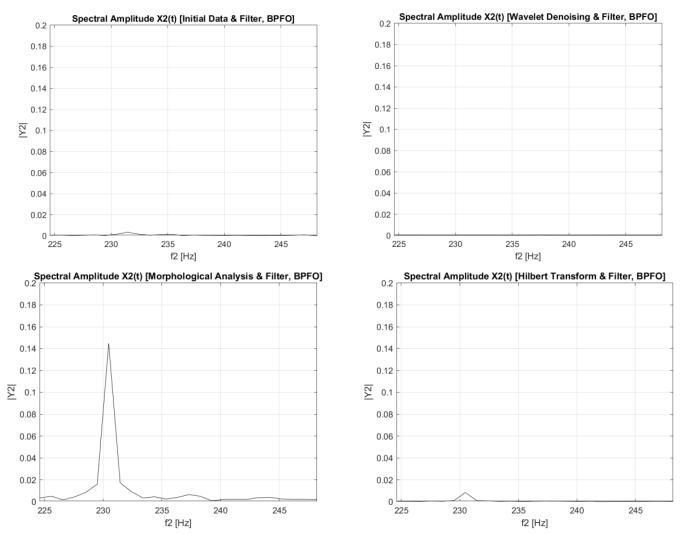





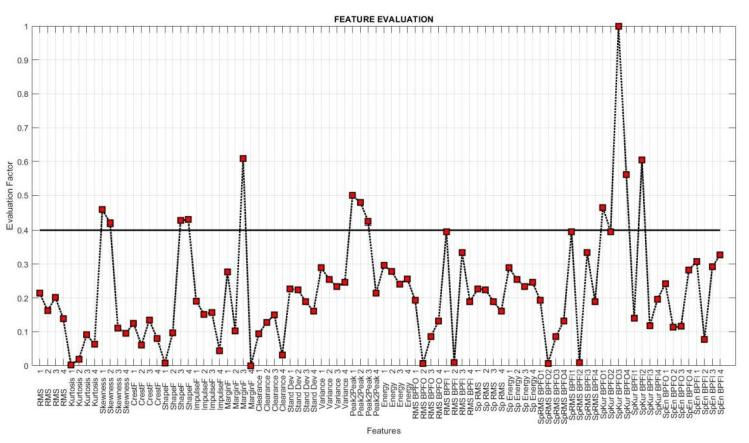





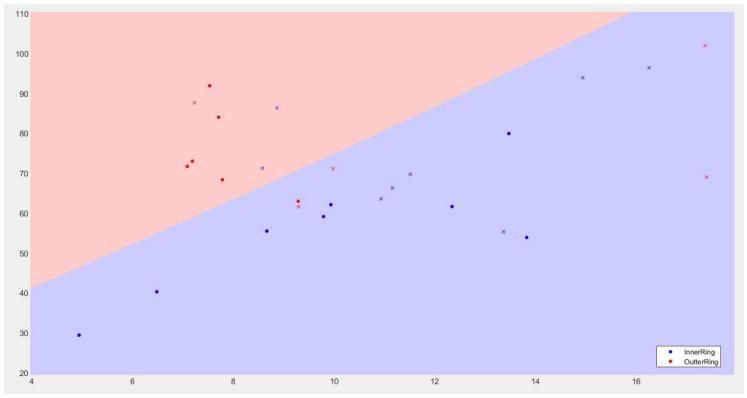



